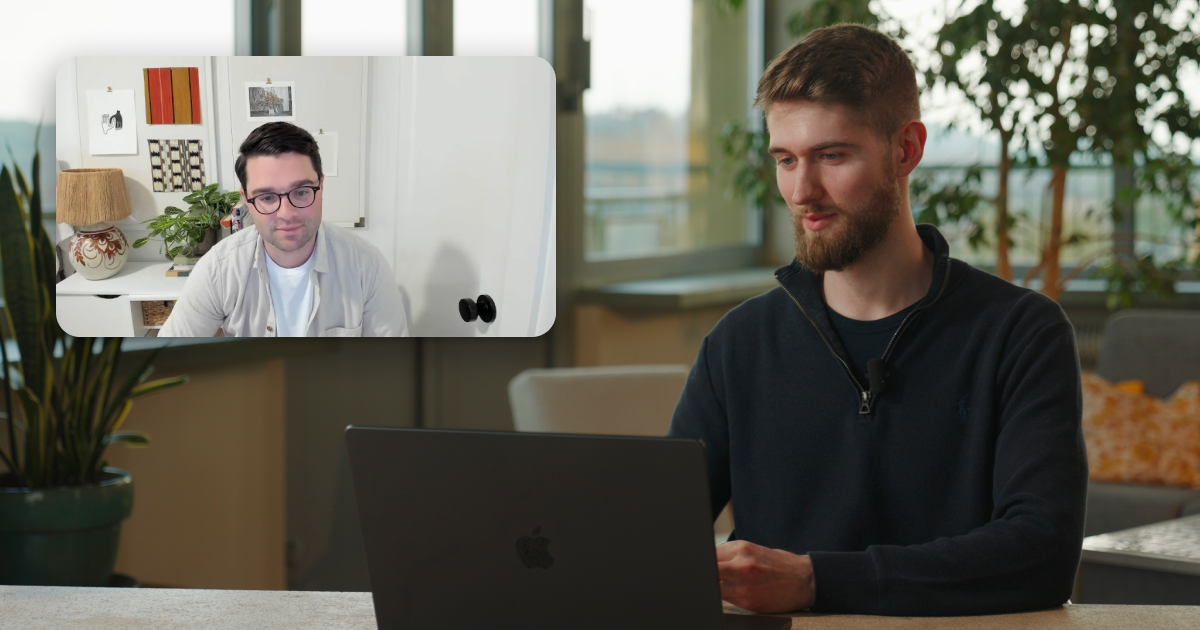
Introduction
I’ve written this article based on my recent conversation with Nick - the Co-Founder and CEO of Seam AI - a customer data SaaS on a mission to democratize the Modern Data Stack. Seam gives business users the power of data analysts without writing SQL. Operations teams leverage Seam to retrieve, transform, analyze, and activate relevant data from a central, simple to use natural language interface.
The problem space
Modern tech companies collect vast amounts of data. The sheer volume can quickly become overwhelming - that’s why they all use what’s called the Modern Data Stack - a suite of cloud tools that aim to gather, store, transform, and analyze their data efficiently. There’s only one problem… Because that data is scattered across dozens, or even hundreds of sources, and dozens of tools, it’s virtually impossible to access the truth without some knowledge of SQL. Most business users (think sales, revenue, or marketing operations) can’t leverage that data independently, which costs fast-growing companies millions of dollars in potential revenue.
Nick - the founder of Seam, has noticed a problem working on the Revenue Operations team at Okta - a billion dollar identity verification SaaS company. Nick experienced the company going through rapid growth phase as it scaled from $100M to $1B in revenue. This growth spike forced Okta to hire more analysts and engineers and build out a complex data system comprised of dozens of tools.
Accessing relevant data quickly became a frustrating task forcing Nick to transition into data analysis role. His dedication proving how bad this problem realy is. That’s how the idea to build an AI interface into customer data was born.
Seam allows companies to connect all sales, marketing, finance an customer success applications into a centralized interface, then analyze relevant data using artificial intelligence. New insights can then be synced to downstream systems. You can think of Seam as the single interface on top of all customer data, enabling retrieval and complex analysis through natural language. Instead of being just another tool in the stack, it bridges the gap between business users and data, unlocking endless revenue opportunities.
100s of interviews and cold outreach through selling
It’s no secret that talking to customers early is the best way to build a great product. Even if you, as the Founder have a solid understanding of the problem space you are largely operating on assumptions before confronting your solution with the market.
Seam took things a step further and conducted initial research by attempting to sell the software. Nick had over a hundred conversations with operations representatives at fast-growing companies to gauge initial interest for a solution like Seam. From there he gradually built relationships and held customer discovery sessions with prospects. All this with just an idea and a PitchDeck. As the team learned more and more about their users they’ve built a front-end demo of the product on Vercel and kept iterating with potential customers.
Soon, their clarity around the solution grew strong and they’d decided to build the backend for their solution. This, as Nick has put it “crawl, walk, run” approach has paid off and let to Seam raising a $5M seed (Link to Seam’s blog) from Bessemer Venture Partners and others.
Learnings along the way - incorrect assumptions
Back when Nick, Mikhiel, and José started working on Seam OpenAI has disrupted the market by releasing ChatGPT to the public. The power of Large Language Models suddenly became available to anyone. Natural language became the language to the computer opening the door to new possibilities across industries. Nick and the team saw this as the perfect opportunity for Seam. With the power of an LLM users could be able to do “self-serve analytics” without needing to have any prior knowledge of data analysis.
The initial idea for the product focused on connecting to user’s data systems, allowing them to perform queries through natural language, and visualize insights. It seemed logical, but the more Nick had tested his prototype with potential customers the clearer it became that they weren’t looking for yet another visualization tool.
Based on customer feedback Seam has pivoted rapidly and instead of trying to build an all-encompassing data analysis tool they’ve decided to help companies operationalize the data tools they were already using. Users can still query all of their data sources with natural language, but instead of visualizing them in Seam they can now easily sync the analyzed data back to the original sources replacing the need for a reverse ETL.
Thanks to utilizing this approach technical teams continue interacting with familiar systems, without the need to alter their existing stack. This shortens the implementation time, and makes onboarding a breeze. At the same time business users gain data superpowers previously reserved for data analysts and engineers.
Founder-market fit - Nick’s bg in Okta
Nick’s frustration with data inaccesibility was his advantage when talking to potential customers. He understood their pain having lived through it at his previous company - Okta. Since his daily tasks as Revenue Operations Representative revolved around supporting sales, marketing and customer success teams from analytics and strategy standpoint he was able to see friction across multiple departments. As the company grew quickly the number of sales and marketing systems exploded from just one tool to over thirty. Okta’s customer data was split across multiple tools, which forced the company to establish a dedicated data team with multiple technical heads and centralize all data into a data warehouse (Snowflake). While it helped the engineering and analytics teams to make sense of the enormous amounts of data the company collected, business users who didn’t know SQL were excluded.
The frustration Nick experienced was so deep that it drove him to learn SQL and transition into a data analytics role. Having seen both sides of the problem - as a business and technical user he embarked on a mission to make the Modern Data Stack accessible to everyone.
What does it mean to NOT solve this problem
The mission behind providing equal access isn’t the only reason for companies to care about this problem. Cutting of potentially hundreds of business users from the data they need has serious negative implications on the bottom line. Nick confidently states that every day that companies choose to ignore this problem is a day they’re missing out on opportunities to be more successful.
Breaking down silos can be expensive and often requires further hires and tooling, not to mention the time spent on implementation which can last multiple months. Companies are also constantly testing and bringing on new systems making it nearly impossible to keep everyone on the same page.
The Modern Data Stack promised to make data more accessible, but because systems have gotten more complex over time implementing them is no longer enough to create a data structure that propels all teams forward. That’s where a tool like Seam comes in. It’s constructed to be stack-agnostic, being able to connect to any combination of popular sources, and tools (replacing some of them; like data transformation, or reverse ETLs). On one hand it unlocks access to data for large numbers of users across the company, on the other - it allows technical teams to maintain their existing data structure, shortening the implementation to days instead of months.
Learnings from the journey to PMF
Seam is getting closer product-market-fit, and although it hasn’t quite reached that milestone yet, the team has seen a great traction with fast-growing companies already (more on that later in the article). I’ve asked Nick about his learnings from the journey and things that in hindsight he would’ve done differently. Here are they key points he highlighted:
- Better customer segmentation
Not all customer feedback is created equal. Back when Nick started talking to customer he would speak with prospects at companies from Series A startups to Enterprises. Although technically they all fell under that same user persona, the problems they were facing were drastically different depending on the scale of their organization. Instead of talking to users with varying needs it would’ve been significantly more productive to concentrate on one, specific type of customer and only move to another group after gaining a deep understanding of the problems prevalent in the first one. - Iterating faster
Ideas - no matter how genius they seem, are typically flawed. The best way to expose and correct these flaws early on is to actively seek external feedback. Delaying that exposure is likely going to lead to time and energy wasted. Having a chance to go through the early stages of product validation again, Nick would’ve tested ideas earlier and dumped the ones that didn’t work without hesitation. - Adapting to the market better
Developing products in a rapidly changing landscape like artificial intelligence coms with an abundance of challenges. Founders need to have a long term vision, but also stay narrowly focused on what the market is saying at every given moment. Finding a way to adapt to both is a constant challenge, but being more aware of it increases the chances of success.
What is ahead of Seam?
With a product ready for scale and a recent public launch Seam is just getting started. During the initial problem exploration in the first year of operations the team have learned a lot about their prospective customers, their needs, and got a solid idea about solutions to their most burning problems. Nick refers to his first year as going from zero to one. Figuring out the exact value proposition, digging deep into customer interviews, and continuously iterating on their prototype allowed his company to attract first customers, and provide tangible value. This year Seam is ready to go from one to a hundred. The company’s “what”, “who”, and “why” is now solidified to a point where it makes sense to scale more aggressively, take big risks and double down on what has worked so far. Seam’s confidence in the product after working with us allowed them to enter customer acquisition mode.
Traction with customers
The team has been working with fast-growing teams at companies like Redpanda (a Series C streaming data platform from San Francisco) and Apploi (a Series B workforce management platform from New York) to unlock their data for business users and save hundreds of hours per year.
In Redpanda’s case, customer data was siloed across multiple systems. Access and reporting were a nightmare for business users and required manual processes that were time-consuming and non-scalable. Seam provided a flexible and scalable solution to track attributions and identify new pipeline opportunities. The growth team also incorporated more buying signals in their account-based marketing strategy based on product usage data, all with a minimal, two-day onboarding process. Thanks to Seam, Redpanda saved hundreds of thousands of dollars across multiple tools and more than 720 hours annually on manual data work (Read the case study on Seam’s website).
- 60 hours a month saved
Redpanda reduced the team’s time spent on manual data work by 60 hours per month (or more than 700 hours annually). - $100,000 saved on tools
Because Seam replaces multiple data-related use cases, Redpanda was able to save six figures per year in tooling alone by building solutions in Seam.
Apploi - another one of Seam’s customers, was also able to achieve great results within months of implementing the app in their organization. Before using Seam, their go-to-market data was scattered across multiple systems. The lack of a single source of truth cost them missed revenue, and reporting was almost impossible. To make matters worse, different teams were working on different metrics (with every team favoring the tool they were most familiar with). That led to substantial alignment challenges and decreased confidence in their data, ultimately hindering their ability to grow.
Apploi implemented Seam as its analytics engine on top of the entire GTM stack. The company unlocked customer intelligence that was previously outside of its reach, automated manual processes, and made sure every team was on the same page. This resulted in substantial savings - 328 and 590 hours annually on manual analytics and operations work, respectively. Additionally, their team identified over $31M of new addressable market for sales to target. (Read the case study on Seam’s website).
- 328 hours of analysts' time saved per yearWith Seam, Apploi was able to connect all of its data and start generating customer intelligence automatically, saving hundreds of hours on data analysis annually.
- 590 hours across operations teams saved per yearApploi used Seam to build automation that helped the finance, sales, and customer success teams save 590 hours annually.
- $31M of new market opportunities identified with Seam
The team is very excited about the future they’re now able to build with Seam:
“Today, too many people view artificial intelligence (AI) as another magical technology that’s being put to work with little understanding of how it works. They view AI as special and relegated to experts who have mastered and dazzled us with it. In this environment, AI has taken on an air of mysticism with promises of grandeur, and out of the reach of mere mortals. The truth, of course, is there is no magic to AI.”
Heading 1
Heading 2
Heading 3
Heading 4
Heading 5
Heading 6
Lorem ipsum dolor sit amet, consectetur adipiscing elit, sed do eiusmod tempor incididunt ut labore et dolore magna aliqua. Ut enim ad minim veniam, quis nostrud exercitation ullamco laboris nisi ut aliquip ex ea commodo consequat. Duis aute irure dolor in reprehenderit in voluptate velit esse cillum dolore eu fugiat nulla pariatur.
Block quote
Ordered list
- Item 1
- Item 2
- Item 3
Unordered list
- Item A
- Item B
- Item C
Bold text
Emphasis
Superscript
Subscript